Keynote Speakers
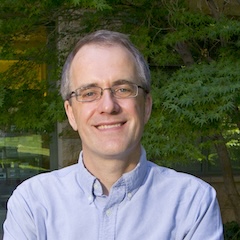
Christopher Manning (Stanford University)
ABSTRACT: Language Models have been around for decades but have suddenly taken the world by storm. In a surprising third act for anyone doing NLP in the 70s, 80s, 90s, or 2000s, in much of the popular media, artificial intelligence is now synonymous with language models. In this talk, I want to take a look backward at where language models came from and why they were so slow to emerge, a look inward to give my thoughts on meaning, intelligence, and what language models understand and know, and a look forward at what we need to build intelligent language-using agents in a world. I will argue that material beyond language is not necessary to having meaning and understanding, but it is very useful in most cases, and that adaptability and learning are vital to intelligence, and so the current strategy of building from huge curated data will not truly get us there, even though LLMs have so many good uses. For a web agent, I look at how it can learn through interactions and make good use of the hierarchical structure of language to make exploration tractable. I will show recent work with Shikhar Murty about how an interaction-first learning approach for web agents can work very effectively, giving gains of 20 percent on MiniWoB++ over either a zero-shot language model agent or an instruction-first fine-tuned agent.
BIO: Christopher Manning is the inaugural Thomas M. Siebel Professor of Machine Learning in the Departments of Linguistics and Computer Science at Stanford University, Director of the Stanford Artificial Intelligence Laboratory (SAIL), and an Associate Director of the Stanford Institute for Human-Centered Artificial Intelligence (HAI). His research goal is computers that can intelligently process, understand, and generate human languages. Manning was an early leader in applying Deep Learning to Natural Language Processing (NLP), with well-known research on the GloVe model of word vectors, attention, machine translation, question answering, self-supervised model pre-training, tree-recursive neural networks, machine reasoning, dependency parsing, sentiment analysis, and summarization. Manning has authored leading textbooks on statistical approaches to NLP and information retrieval, linguistic monographs on ergativity and complex predicates, and the CS224N Natural Language Processing with Deep Learning course, available on YouTube. He is an ACM Fellow, a AAAI Fellow, and an ACL Fellow, and a Past President of the ACL (2015). His research has won ACL, Coling, EMNLP, and CHI Best Paper Awards, an ACL Test of Time Award, and the IEEE John von Neumann Medal (2024). He is the founder of the Stanford NLP group (@stanfordnlp) and manages development of the Stanford CoreNLP and Stanza software.
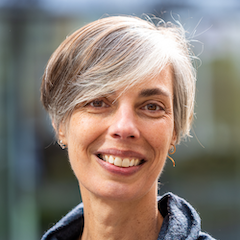
Raquel Fernández (University of Amsterdam)
ABSTRACT: Large language models have opened up new scientific opportunities in a variety of fields. Some challenges that had been core roadblocks in natural language processing for decades – such as inferring rich representations for language understanding and generating fluent and complex text – have now mostly been overcome. An exciting consequence of this is that we can now dive into more nuanced problems within the language sciences. Two frontiers in the computational modelling of language use – which we should arguably be better equipped to tackle now – concern perceptual grounding and social interaction. In this talk, I will review some of the current challenges of language models regarding these frontiers, present some recent work on multimodal and conversational grounding, and argue that there is still substantial progress to be made and plenty of interesting open questions ahead of us.
BIO: Raquel Fernández is Full Professor of Computational Linguistics and Dialogue Systems at the Institute for Logic, Language & Computation, University of Amsterdam, where she leads the Dialogue Modelling Group. Her work and interests revolve around language use in context, including computational semantics and pragmatics, dialogue interaction, visually-grounded language processing, and language learning, among others. Her group carries out research on these topics at the interface of computational linguistics, cognitive science, and artificial intelligence. Raquel studied language and cognitive science in Barcelona, her home city, and received her PhD in computational linguistics from King's College London. Before moving to Amsterdam, she held research positions at the Linguistics Department of the University of Potsdam and at the Center for the Study of Language and Information (CSLI), Stanford University. Over the course of her career, she has been awarded several prestigious personal fellowships by the Dutch Research Council and is the recipient of a European Research Council (ERC) Consolidator Grant.
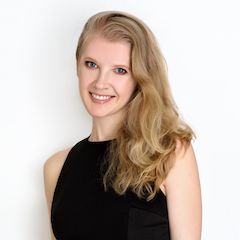
Evelina Fedorenko (MIT)
ABSTRACT: I seek to understand how our brains understand and produce language. Patient investigations and neuroimaging studies have delineated a network of left frontal and temporal brain areas that support language processing, and work in my group has established that this “language network” is robustly dissociated from both lower-level speech perception and articulation mechanisms, and from systems of knowledge and reasoning (Fedorenko et al. 2024a Nat Rev Neurosci; Fedorenko et al., 2024b Nature). The areas of the language network appear to support computations related to lexical access, syntactic structure building, and semantic composition, and the processing of individual word meanings and combinatorial linguistic processing are not segregated spatially: every language area is sensitive to both (e.g., Shain, Kean et al., 2024 JOCN). In spite of substantial progress in our understanding of the human language system, the precise computations that underlie our ability to extract meaning from word sequences have remained out of reach, in large part due to the limitations of human neuroscience approaches. But a real revolution happened a few years ago: a candidate model organism emerged, albeit not a biological one, for the study of language—neural network language models (LMs), such as GPT-2 and its successors. These models exhibited human-level performance on diverse language tasks, including those long argued to only be solvable by humans, and often producing human-like output. Inspired by the LMs’ linguistic prowess, we tested whether the internal representations of these models are similar to the representations in the human brain when processing the same linguistic inputs, and found that indeed LM representations predict neural responses in the human language areas (Schrimpf et al. 2021 PNAS). This model-to-brain representational similarity opens a lot of exciting doors to investigations of human language processing mechanisms (for a review see Tuckute et al., 2024 Ann Rev Neurosci). I will discuss several lines of recent and ongoing work, including a demonstration that LMs align to brains even after a relatively small amount of training (Hosseini et al., 2024 Neurobio of Lang), a closed-loop neuromodulation approach to identify the linguistic features that most strongly drive the language system (Tuckute et al., 2024 Nat Hum Beh), and work on the universality of representations across LMs and between LMs and brains (Hosseini et al., in prep.).
BIO: Dr. Fedorenko is a cognitive neuroscientist who studies the human language system and its relationship with other systems in the brain. She received her Bachelor’s degree from Harvard University in 2002, and her Ph.D. from MIT in 2007. She was then awarded a K99R00 Pathway to Independence Career Development Award from NIH. In 2014, she joined the faculty at MGH/HMS, and in 2019 she returned to MIT where she is currently an Associate Professor in the Department of Brain and Cognitive Sciences and a member of the McGovern Institute for Brain Research. Dr. Fedorenko uses fMRI, intracranial recordings and stimulation, EEG, MEG, and computational modeling, to study adults and children, including individuals with developmental and acquired brain disorders, and individuals with structurally atypical brains, but typical-like cognition.
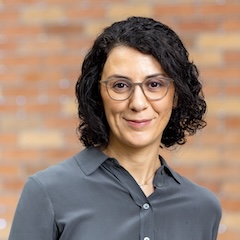
Hannaneh Hajishirzi (University of Washington / AI2)
ABSTRACT: Language models (LMs) have become ubiquitous in both AI research and commercial product offerings. As their commercial importance has surged, the most powerful models have become closed off, gated behind proprietary interfaces, with important details of their training data, architectures, and development undisclosed. Given the significance of these details in scientifically studying these models, including their biases and potential risks, I argue that it is essential for the research community to have access to powerful, truly open LMs. In this talk, I present our OLMo project aimed at building strong language models and making them fully accessible to researchers along with open-source code for data, training, and inference. I describe our efforts in building language modeling from scratch, expanding their scope to make them applicable and useful for real-world applications, and investigating a new generation of LMs that address fundamental challenges inherent in current models.
BIO: Hanna Hajishirzi is the Torode Family Associate Professor in the Allen School of Computer Science and Engineering at the University of Washington and a Senior Director of NLP at AI2. Her current research delves into various domains within Natural Language Processing (NLP) and Artificial Intelligence (AI), with a particular emphasis on accelerating the science of language modeling, broadening their scope, and enhancing their applicability and usefulness for human lives. She has published over 140 scientific articles in prestigious journals and conferences across ML, AI, NLP, and Computer Vision. She is the recipient of numerous awards, including the Sloan Fellowship, NSF CAREER Award, Intel Rising Star Award, Allen Distinguished Investigator Award, Academic Achievement UIUC Alumni Award, and was a finalist for the Innovator of the Year Award by GeekWire. The work from her lab has been nominated for or has received best paper awards at various conferences and has been featured in numerous magazines and newspapers.